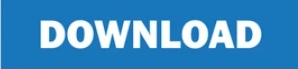
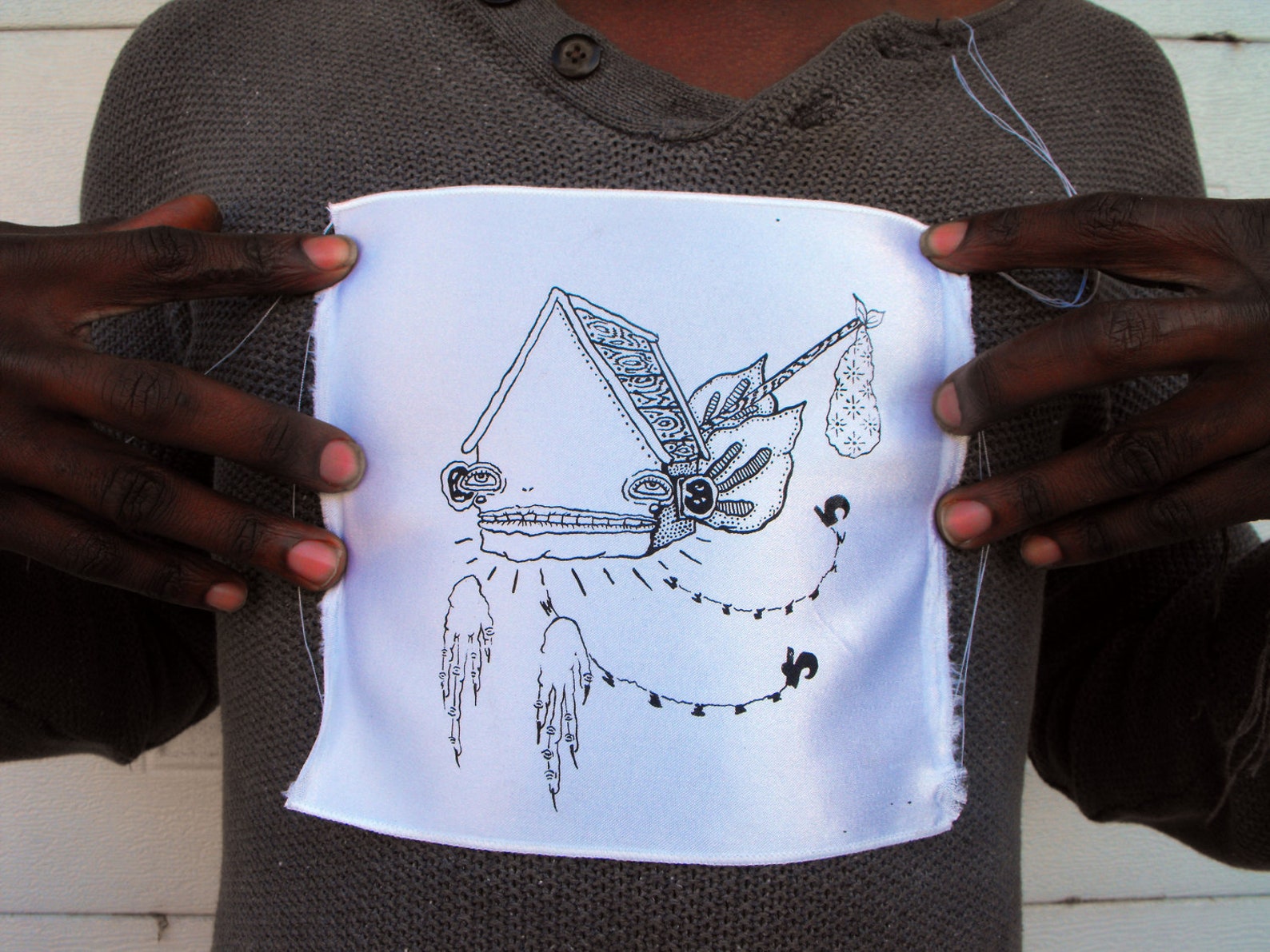
Our research alerts AI practitioners that validating the model based only on the metrics may lead to a wrong conclusion. With the detailed analysis of the result, we have found some cases that revealed the ambiguity of the decisions caused by the inconsistency in data annotation. However, the data pre-processing techniques deteriorated the metrics used to measure the overall performance, such as F1-score and Average Precision (AP), even though we empirically confirmed that the malfunctions improved.

These results support that the data-centric approach might improve the detection rate. We found that CLAHE increased Recall by 0.1 at the image level, and both CLAHE and BR improved Precision by 0.04–0.06 at the bounding box level. We have experimented with analyzing the effect of the methods on the inspection with the self-created dataset. We used a data-centric approach to solve the problem by using two different data pre-processing methods, the Background Removal (BR) and Contrast Limited Adaptive Histogram Equalization (CLAHE).
#Silkscreen patch trial
During the on-site trial test, we experienced some False-Positive (FP) cases and found a possible Type I error. In this paper, we propose an inspection system based on deep learning for a tampon applicator producer that uses the applicator’s structural characteristics for data acquisition and uses state-of-the-art models for object detection and instance segmentation, YOLOv4 and YOLACT for feature extraction, respectively.
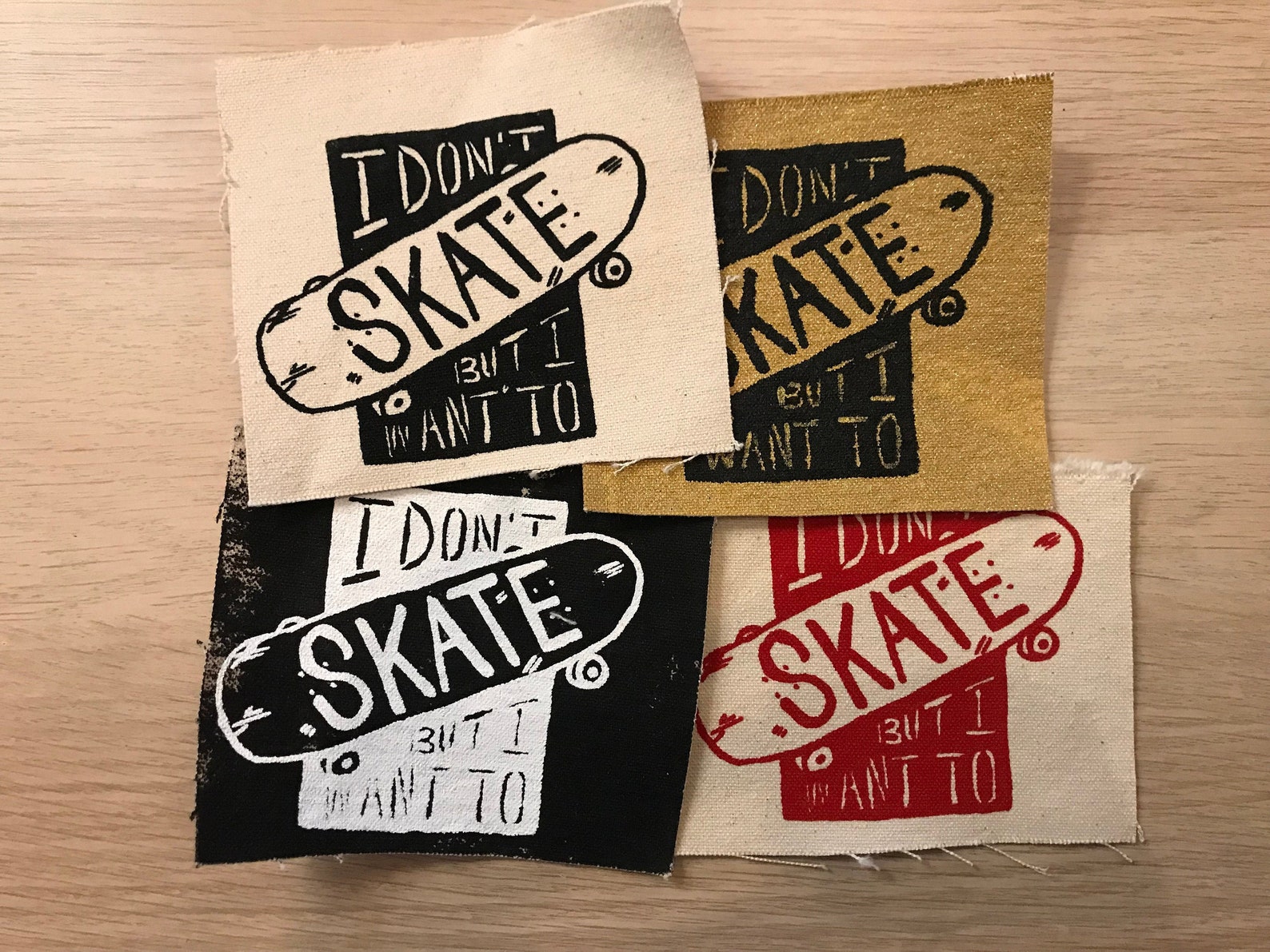
However, some manufacturers have failed to apply the traditional vision system because of constraints in data acquisition and feature extraction. Manufacturers are eager to replace the human inspector with automatic inspection systems to improve the competitive advantage by means of quality. The present work provides an effective and efficient framework to detect different growth stages under a complex orchard scenario and can be extended to different fruit and crop detection, disease detection, and different automated agricultural applications. The proposed Dense-YOLOv4 has outperformed the state-of-the-art YOLOv4 with 7.94%,13.10%,10.47%, and 4.73% increase in precision, recall, F1-score, and mAP, respectively. At a detection rate of 44.2 FPS, the mean average precision (mAP) and F1-score of the proposed model have reached up to 96.20% and 93.61%, respectively. The model has been applied to detect different growth stages of mango with high degree of occultation in a complex orchard scenario. Furthermore, a modified path aggregation network (PANet) has been implemented to preserve fine-grain localized information. The current work proposes a real-time object detection framework Dense-YOLOv4 based on an improved version of the YOLOv4 algorithm by including DenseNet in the backbone to optimize feature transfer and reuse. However, due to considerable degree of occultation in surrounding leaves, significant overlapping between neighboring fruits, differences in size, color, cluster density, and other growth characteristics, traditional detection methods have the limitation in the accuracy of detecting different growth phases. Real-time detection of agricultural growth stages is one of the key steps of estimating yield and intelligent spraying in commercial orchards. In addition, it was shown that quality inspection using the patch-split method-based AI is possible even in situations where there are few prior defective data. All of the fine defects in products, such as silk screen prints, could be detected regardless of the product size. The proposed model was applied to an actual silk screen printing process. In the existing technique with the original image as input, artificial intelligence (AI) learning is not utilized efficiently, whereas our proposed method learns stably, and the Dice score was 0.728, which is approximately 10% higher than the existing method.
#Silkscreen patch Patch
The novelty of the method is that, to better handle defects within an image, patch level inputs are considered instead of using the original image as input. In this paper, an improved U-Net++ is proposed based on patch splits for automated quality inspection of small or tiny defects, hereinafter referred to as ‘fine’ defects. The types and locations of defects that usually occur in silk screen prints may vary greatly and thus, it is difficult for operators to conduct quality inspections for minuscule defects. The trend of multi-variety production is leading to a change in the product type of silk screen prints produced at short intervals.
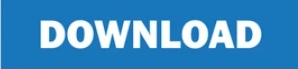